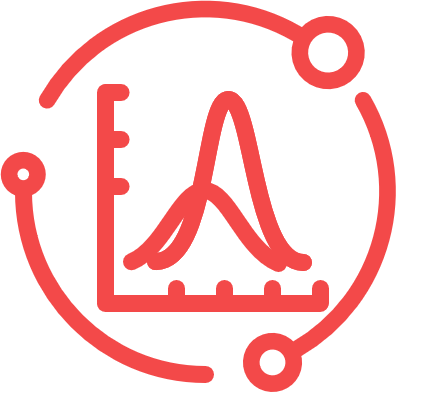
MULTI SCALE PERFORMANCE MODELLING
The vision of CerTest is realised through four flexible and highly interlinked and interdependent Research Challenges (RC1‐RC4), utilising a multiscale approach drawing on the expertise highlighted by the Themes (T1‐T3).
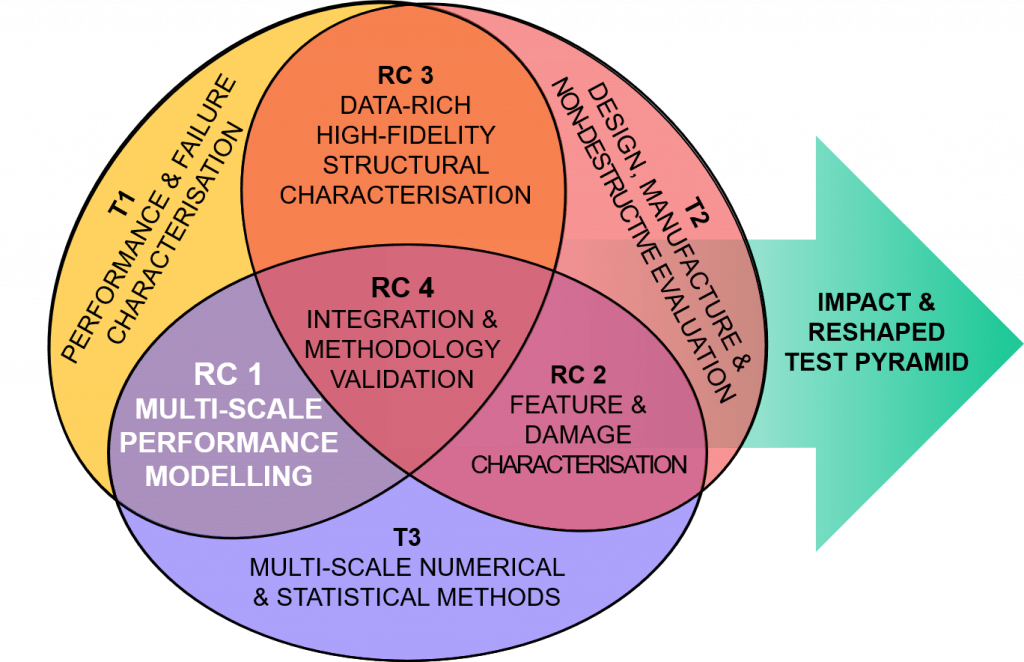

Fig. 1 Interconnection of Research Challenges (RCs) and Themes (Ts) leading to impact.
In this research challenge, a multi‐scale statistical modelling framework will be developed and validated that incorporates:
BAYESIAN STATISTICS
MODEL ORDER REDUCTION TECHNIQUES
ITERATIVE SOLVERS
These will predict load response, damage initiation and progression in complex composite components and structures, including the impact of as‐designed subsurface features, manufacturing variability, damage and their statistical distributions. Particular attention will be paid to creating new modelling capability at the component‐level that can predict structural (macroscale) and material (meso‐scale) failure whilst accounting for statistical variation in manufacturing defects, material properties, operational environment, impact damage and lifetime loading .
Multi‐scale high‐fidelity image‐based experimental data, gathered in RC2 and RC3, will be integrated into the Bayesian description of model data in RC1. Meso‐scale data (from RC2) relating to geometry of as‐designed features, damage and defect morphology will be combined with meso‐scale failure test data to guide and inform model development and validation. The stresses and strains derived from the data‐rich images from the structural tests over a range of scales (RC3) will provide input for calibration/validation at the macro‐scale, both pre and post failure. The new numerical models will be used to implement the statistical models developed in RC1 for the integration of all these aspects in a Bayesian Design of Experiments (DoE) and for Bayesian updating of the relevant posterior distributions in RC4 to reduce uncertainty and to increase confidence.

New multi‐scale statistical modelling frameworks, including use of novel iterative solvers, model order reduction and damage models, will allow for the effect of generalised micro/meso features and damage to be captured at the structural scale.
Background and Motivation
Mechanical modelling of aerospace structures offers a complex and diverse set of challenges stemming from the often‐competing requirements of design, analysis and certification, as well as, the varying operating environments (eg. engine component vs wing structure).
Most virtual test methods focus on simulation using complex modelling of delamination and ply failure. The process is highly nonlinear and requires explicit tracking of instabilities via time stepping. Comparison with physical testing reveals that stable propagation initiates at stress levels below ultimate design loads, in the vicinity of stress concentrations caused by ‘as‐designed’ features, defects or impact damage. Hence, models require high fidelity to capture rapid variations in stress associated with localised geometric features. The resulting high computational cost restricts the majority of these approaches to coupon‐level simulation.