
INTEGRATION AND METHODOLOGY VALIDATION
The vision of CerTest is realised through four flexible and highly interlinked and interdependent Research Challenges (RC1‐RC4), utilising a multiscale approach drawing on the expertise highlighted by the Themes (T1‐T3).


Fig. 1 Interconnection of Research Challenges (RCs) and Themes (Ts) leading to impact.
RC4 aims to create a step change in integration of data‐rich experimental procedures and predictive statistical and multi‐scale computational models by developing data processing and a closed input/feedback loop based on novel Bayesian Learning and Design of Experiments (DoE) techniques. RC4 is strongly inter‐linked with RC1‐RC3. RC4 will inform data collection strategies in the other RCs, and receive from them: data to inform multi‐scale statistical models (RC1), parametric descriptions and distribution functions of critical features and defects (RC2), and experimental design and performance data (RC3) in a closed input/feedback loop.
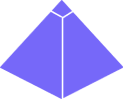
Background and Motivation
RC4 provides the principal route of delivery for the overall vision of the PG. To achieve this, fundamental scientific breakthroughs are required that will be delivered in each of RC1, RC2 and RC3. However, the overarching vision of the PG and truly transformative scientific research on integration of multi‐scale models with test data can only be achieved via addressing the key methodological challenges in RC4. Thus RC4, integrated and inter‐related with RC1‐RC3, is the prerequisite for the development and validation of the envisioned science‐based composite aero‐structure design and verification capability. This in turn provides the foundation for a new science‐based approach at TRL1‐3 to ‘certification for design’ achieved through reshaping and reduction of the conventional ‘testing pyramid’, and hence provides the basis for impact and industrial uptake at TRL4‐6 (see ‘Pathways to Impact’).
The testing pyramid is currently used to prove airworthiness to define the performance structural integrity of composite aero‐structures using a building block approach. The premise is that conservative allowable strength limits of statistically independent and mostly small parts or elements of a composite aero‐structure (mainly coupons) is combined with knock‐down factors to define performance at the higher component/sub‐structure levels. Such a strategy is globally sub‐optimal and leads to inadequate uncertain‐ty quantification, and overly conservative design decisions regarding performance probability of failure. Design freedom is restricted as the procedure does not account for elements that may improve performance but cannot be assessed at coupon level, and the effect of manufacturing features cannot be adequately represented. The outcome is an inefficient structure carrying significant weight penalty with a corresponding increase to materials usage, increased fuel consumption and reduced payload capacity.
RC4 facilitates the new approach to certification by defining the necessary methods and tools for a holistic approach to the fusion of physical and computational data from the view point of decision‐theoretic statistical Design of Experiments (DoE)63. To achieve this, following the principles outlined in WP4.1 below, experimental and computational resource will be allocated to maximally increase information content as predicted by the current state of knowledge derived from the multi‐scale modelling across different physical scales (e.g. meso‐scale features through to macro‐scale composite structures), different data types (e.g. full field imaging data, ultrasound, X‐ray, etc.), and different modelling resolutions. Experimental data provided by RC2 and RC3 will be used in a Bayesian way to calibrate and validate the models in RC1 and used to inform DoE. Hence, modelling will inform and refine data collection in the PG.
RC4 Posters
Research Associates
PhD Students

Thomas Maierhofer
University of Bath